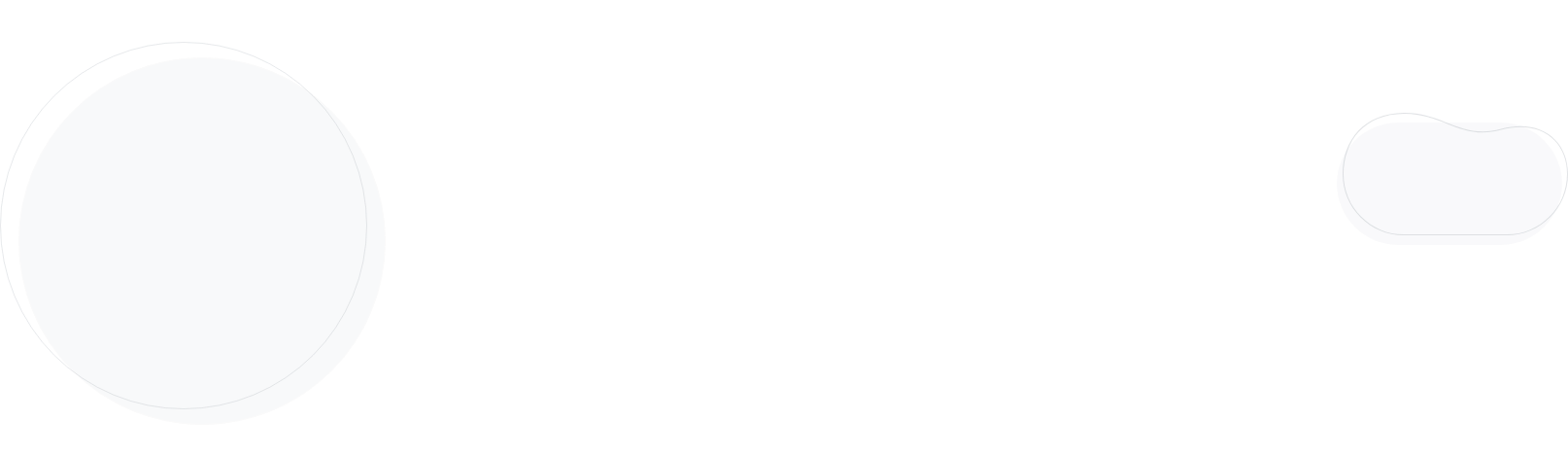
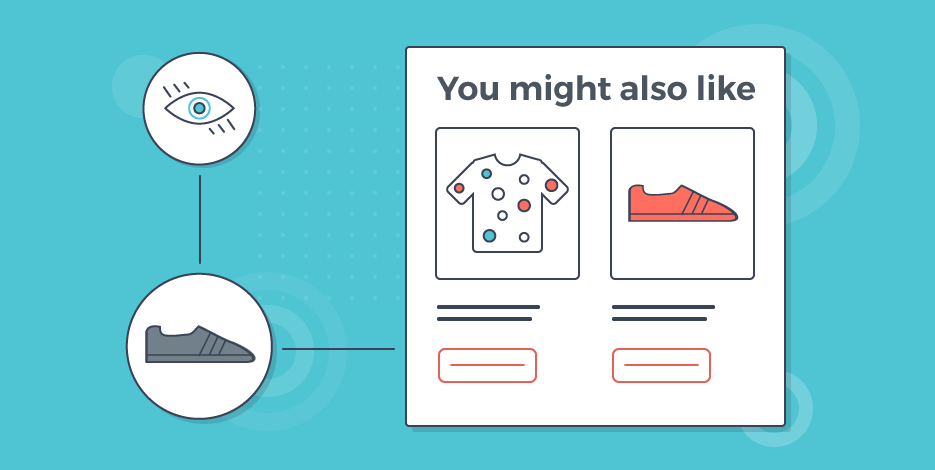
Product Recommendations: Driving Revenue With Our 5-star Feature
We are thrilled to announce the release of the most intelligent Product Recommendation System in the industry! Our Enterprise feature offered as a free trial for 3 whole months on all existing accounts. Yes, we are THAT amazing!
Now all small to medium-sized businesses can rejoice. Because Moosend democratizes access to Big Data and improves your website performance and conversions. Automatically.
Read on to find out more about what this release is all about and what makes it so unique.
What is a Product Recommendation system?
Imagine greeting your first-time customers with your hot sellers. Sending your subscribers personalized deals based on their purchase history and interests. Or informing them of your latest products, items usually purchased together or what other customers with the same tastes have also purchased.
This is what a Product Recommendation system (PRS) is all about. Making your wildest e-shop dreams come true.
In a few words, it’s a business’ way to improve online shopping experiences for customers, provide key metrics for online store owners and drive more sales.
According to a study conducted by Barilliance, shoppers that clicked on recommendations are 4.5x more likely to add items to cart, and 4.5x more likely to complete their purchase.
Usually, such dynamic product recommendations are the big player’s privilege. Because their recommendations are informed from a larger number of user-profiles and behavioral patterns. Hence the higher accuracy.
BUT Moosend’s PRS changes the game by taking small and medium businesses product recommendations on the same level.
Which means that you can now increase your sales by making targeted product recommendations in the fastest and most accurate way, based on users’ previous viewing or shopping behavior. Just like Amazon!
Why is Moosend a pioneer in PRS?
Because we help with the lack of quality or quantity of data to enhance your data sets.
How? By using our huge database, containing millions of rows of processed customer interaction data from businesses around the globe.
In other words, our code trains your model!
You could say it’s a cross-shop “interaction-map”, with customers’ preferences and interests.
And you have all the data knowledge of a “big player”, processed and ready to use in your business.
Getting started
It all starts with our powerful web tracker, Mootracker, which starts collecting your products and stores them to our database as soon as you install it.
Running a rigorous 24/7 monitoring, Mootracker captures everything on your website regarding your products and maps this data with other websites’. This way we derive more data points about the preferences of similar buyers.
This is the baseline of our system!
But how do we use them for perfecting our product recommendations?
With the help of clustering.
It’s a two-step way to heaven.
First, we identify groups of similar products across shops.
Then, we split stores into segments, based on the same or similar products we have already identified in product clustering. For example, all fashion retailers are bundled together in a segment and home retailers in another one.
These 2 methods, product, and store clustering are the absolute success combo. Because they help us gather only the valuable information that makes sense to your store so you learn from the big players the customer purchase patterns that work for you.
Which lets us form the ideal product recommendations for your eyes only!
How the cookie crumbles
There are plenty of recommendation systems for every situation. We use Collaborative Filtering, a system built on the concept that customers with similar buying behavior also share similar interests.
In other words, the good old “People who viewed this product also viewed this”.
How to squeaky-clean the information
Every person is different.
And this is a factor we took into consideration when building our model. Otherwise, it would be doomed to fail!
Confused?
Let’s say that the product “A” appeals to both users James and Nick. And they both give it a score of 3 out of 5. James rates the products with which he interacted with an average score of 2.8, while Nick with an average of 4.
Clearly, the scores for product A are the same. But these mean different things for both.
James is a sophisticated user and has higher standards, therefore he scores the products with lower rates. That being said, a 3 out of 5 review is considered to be a good one for James.
Nick, on the other hand, tends to assign higher rates more easily, therefore his reluctance to do so with the given product suggests that this is a poor performance score.
This difference we just described ladies and gents, is what we call bias.
And because it messes with our product recommendations we had to remove it.
Removing the bias from our interaction terms is a kind of a matrix normalization technique. In that way, we remove personal interests so we can make our unbiased calculations.
Finally, we add the customers extracted interest at the end of the matrix factorization process.
Getting our code to recommend products
When we de-bias our matrix we split it into products and customers, each with their latent factors.
These factors are used to determine the terms in which we describe a product or customer.
For example, let’s say that your shop only sells mobile phones. In this case, a latent factor could refer to either “the software that they use” or “the inches of the screen”.
Ultimately, latent factors help us describe and reveal patterns in a lower-dimensional space, thus estimating the customer-product interest score.
Furthermore, we take into account the special weight of customer behaviors.
For example, a visitor might be browsing skirts for his girlfriend. But what he is really interested in are chinos for himself. Our model is clever enough to know when you are moving closer or further from a good result, helping you get to the appropriate recommendations for each user.
What about Interest Decay?
And then you have some highly time-bound items. Like the champagne glasses one might be looking at for a friend’s wedding. Or the swimsuit they wanted to buy for their summer vacation.
It wasn’t easy. But I won’t go all techy with you now. All you need to know is we finally created the perfect formula so our model can tell how the personal interest changes and what a customer needs at a given moment.
Whatsmore, our formula changes for every store cluster, simply because customers have different buying habits between different shops.
For example, think about the purchasing frequency between a supermarket and a fashion shop. Completely different, right?
Ready to roll
That’s all folks! Our super amazing Product Recommendation system is good to go and we are so proud to share it with you.
Now we invite you to use it (i.e. train it) while we are getting down to business for our next feature.
Until then, cheerio!